LLMs have potential for medical information access but face challenges with citing reliable sources. Enhancing database integration and source attribution is key to improving their accuracy.
However, one of the critical questions for anyone using LLMs for medical purposes is: How well do these models cite relevant and accurate medical information?
The Role of Reliable Citations in Medicine:
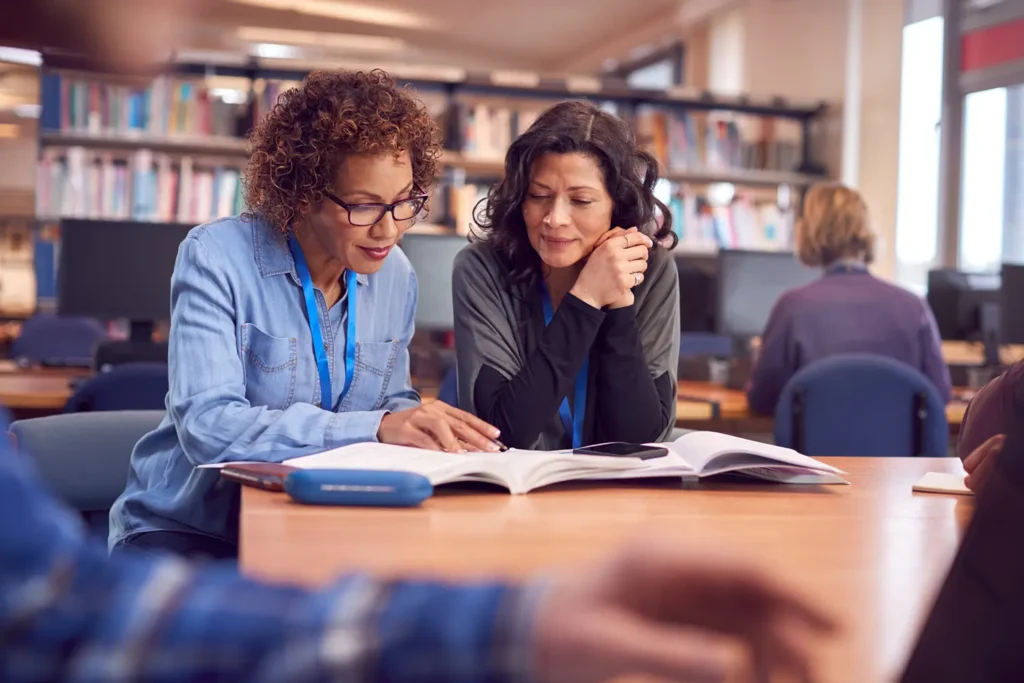
In the medical field, where people’s health and lives are at stake, the quality and accuracy of information are essential. Doctors, researchers, and patients all rely on up-to-date, evidence-based information to make informed decisions. This is why citation from reliable sources such as clinical trials, peer-reviewed journals, and reputable health organizations is crucial when discussing medical topics.
Without proper citations, medical information can be deemed untrustworthy, and using incorrect or outdated data can have severe consequences. Therefore, it’s essential to understand the limitations and capabilities of LLMs when it comes to citing relevant medical sources.
How LLMs Handle Medical Data:
LLMs, by their nature, are designed to process vast amounts of data and generate text based on patterns in that data. They are trained on a wide range of text sources, which may include books, websites, research papers, and other publicly available documents. However, LLMs do not have direct access to proprietary or real-time medical databases like PubMed, ClinicalTrials.gov, or similar resources. Instead, they generate answers based on the data they’ve ingested during their training process, which can include both authoritative and unreliable sources.
This lack of access to real-time medical data is a significant challenge when it comes to providing up-to-date and fully accurate medical information.
Here are some ways LLMs might fall short:
Outdated Information:
Since LLMs do not update their knowledge after their training period, they may provide outdated medical information, missing the latest research, guidelines, or treatments. Medical knowledge evolves rapidly, and what was once considered best practice can change over time.
Lack of Source Attribution:
One of the most significant drawbacks of LLMs is their inability to cite sources. When responding to medical queries, LLMs may generate useful insights, but without the ability to point to the original sources, users cannot easily verify the credibility or accuracy of the information. In medical practice, this lack of citation makes the information less trustworthy and potentially risky to rely on.
Contextual Relevance and Specificity:
While LLMs are good at processing general medical information, they can sometimes provide responses that are only somewhat related to a user’s query. For example, a query about a specific disease in a particular population might receive a generalized response based on broader knowledge rather than the most contextually relevant data. This can lead to answers that, while not necessarily wrong, may not fully meet the specific needs of the user.
Bias in Training Data:
Like any machine learning model, LLMs are only as good as the data they are trained on. If the training data contains biased or incomplete information, it can reflect those biases in the model’s responses. In medicine, this could result in recommendations that are skewed or based on outdated practices, especially when the data used to train the model is not representative of the latest scientific consensus.
Also Read: How Long Does Certification For Medical Assistant Take – Fast-Track Options and Time Estimates”!
How Can LLMs Improve in Citing Medical Information?
Given the challenges above, several improvements could be made to enhance how LLMs handle and cite medical information:
Integration with Trusted Medical Databases:
A major leap forward would be the integration of LLMs with trusted medical databases such as PubMed, ClinicalTrials.gov, and others. These databases house the most current, peer-reviewed research and guidelines. By incorporating real-time data from these sources, LLMs would be able to provide answers based on the most up-to-date and reliable evidence, reducing the risk of outdated or incorrect medical advice.
Explicit Source Attribution:
One of the most important changes LLMs could undergo is the ability to explicitly cite their sources. This would allow users to trace the information back to its original reference, which is crucial for medical contexts. Incorporating proper citations into responses could significantly increase the credibility of the information provided by LLMs and ensure transparency in the sources used to generate responses.
Fine-Tuning with Medical Data:
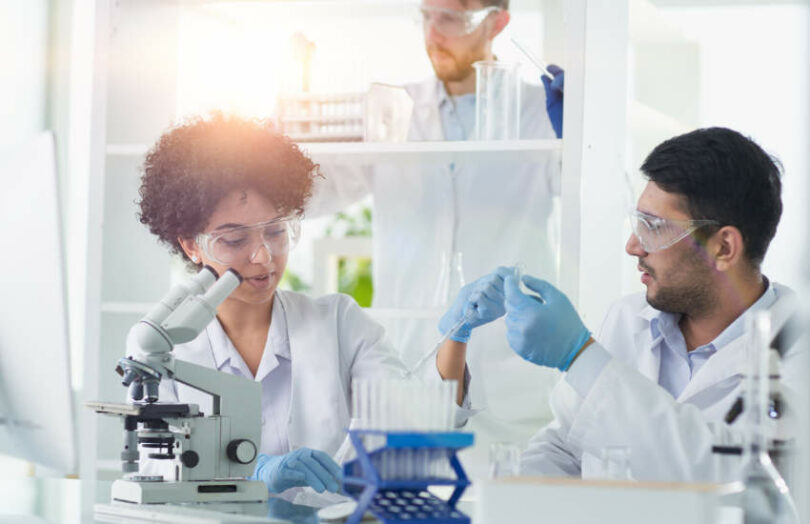
While LLMs are trained on a broad set of texts, they can be fine-tuned using specialized medical datasets. By training models specifically on high-quality, peer-reviewed medical research, medical textbooks, and authoritative health resources, the model’s accuracy in medical contexts could be greatly improved. Fine-tuning could also help LLMs better understand the specific language and nuances used in medical discussions, leading to more accurate and contextually appropriate responses.
Real-Time Updating:
Since medical information evolves rapidly, the ideal scenario would involve LLMs being able to continuously update their knowledge base to reflect the most recent research. This would mean regularly retraining the model with the latest medical studies, guidelines, and discoveries. Incorporating such updates could help bridge the gap between traditional static models and the dynamic nature of medical science.
Collaboration with Healthcare Professionals:
Another way to improve the reliability of medical information provided by LLMs is through collaboration with healthcare professionals. By working alongside doctors, researchers, and other medical experts, LLMs could receive additional oversight and validation for their outputs. This collaboration could ensure that the medical advice generated by the model is not only relevant but also safe and appropriate.
Challenges in Using LLMs for Medical Advice:
While LLMs can be helpful for generating basic information and answering general medical queries, they still face several challenges when used for more complex or critical medical advice:
- Lack of Personalization: LLMs provide generalized information based on patterns and data they’ve seen during training. However, medical treatment is highly individualized. A model may not be able to fully account for a person’s unique medical history, genetics, or other factors that influence health outcomes.
- Potential for Misinterpretation: Medical topics often involve nuanced details that may be difficult for a language model to interpret correctly. The model might over-simplify complex medical conditions or fail to explain the risks associated with certain treatments adequately.
- Ethical Concerns: There are also ethical issues surrounding the use of LLMs for medical advice. Because these models don’t have true understanding or consciousness, they may inadvertently provide harmful or misleading information. This underscores the importance of human oversight when relying on LLMs in medical settings.
FAQ’s
1. How do LLMs handle medical data?
LLMs process vast amounts of data but don’t access real-time medical databases, which may result in outdated or unreliable information.
2. Why are citations important in medical information?
Citations ensure that medical data is credible, accurate, and traceable, making it essential for informed healthcare decisions.
3. What are the main limitations of LLMs in citing medical information?
LLMs cannot cite sources, making it hard to verify the credibility or accuracy of their responses.
4. How can LLMs improve in medical information accuracy?
Improvements include integrating with trusted medical databases, explicit source attribution, and fine-tuning with medical datasets.
5. Can LLMs replace healthcare professionals?
No, LLMs can complement but should not replace professional medical advice due to limitations in personalization and accuracy.
Conclusion
LLMs offer great potential for transforming medical information access, but their ability to cite reliable sources remains a challenge. While they provide general insights, users must verify this information with trusted sources. To become truly effective in healthcare, LLMs need improvements such as better database integration, explicit citations, and regular updates. They can complement, not replace, professional advice.
Leave a Reply